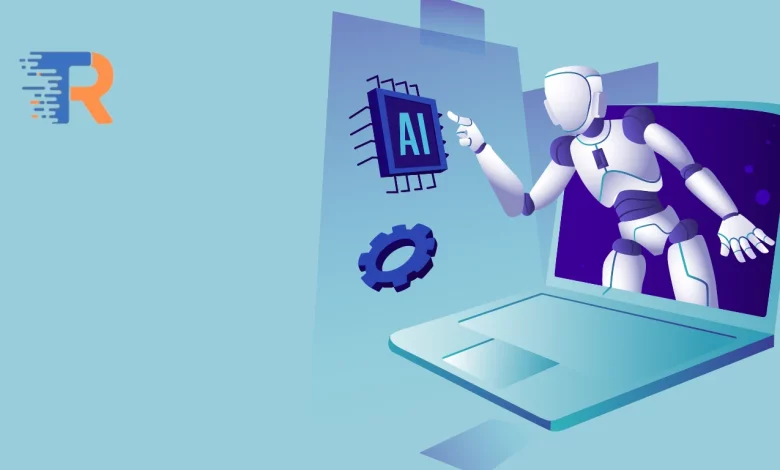
In the realm of AI Models Support Robots, the challenge lies not just in systematizing intricate tasks but in infusing machines with the ability to understand and perform nuanced instructions similar to human insight. As everyday chores seamlessly unfold for individuals, each step in the process becomes intuitive. This poses a unique set of challenges for robots, as they require intricate planning and detailed outlines to navigate and execute even seemingly simple tasks.
Enter HiP, a revolutionary approach that diverges from traditional multimodal models by utilizing three distinct foundation models. Unlike its counterparts, HiP negates the need for paired vision, language, and action data, making the reasoning process more transparent and efficient. Let’s delve into the key aspects of HiP and its groundbreaking approach to imbuing robots with linguistic, physical, and environmental intelligence.
Read More: ABB Elevates Mobile Robots
- Unlike RT2 and other multimodal models trained on paired vision, language, and action data, HiP employs three distinct foundation models, each trained on different data modalities. These models capture various aspects of the decision-making process and collaborate when making decisions, eliminating the need for access to challenging-to-obtain paired vision, language, and action data.
- Your daily to-do list comprises simple tasks like washing dishes and buying groceries, but the intricate steps involved in these chores, such as “pick up the first dirty dish” or “wash that plate with a sponge,” are often intuitive for humans.
- HiP streamlines the reasoning process and enhances transparency, making it a unique solution in contrast to monolithic foundation models like RT2. This approach addresses the challenges of pairing language, visual, and action data, which can be expensive and difficult to obtain.
- What may seem like a routine daily chore for a human constitutes a “long-horizon goal” for a robot. This overarching objective involves completing numerous smaller steps, necessitating ample data for planning, understanding, and executing objectives.
- Computer vision researchers have traditionally attempted to construct monolithic foundation models to tackle this issue. However, HiP introduces a distinct multimodal recipe that efficiently incorporates linguistic, physical, and environmental intelligence into a robot, providing a cost-effective and comprehensive solution.
- Unlike RT2 and other multimodal models trained on paired vision, language, and action data, HiP employs three distinct foundation models, each trained on different data modalities. These models capture various aspects of the decision-making process and collaborate when making decisions, eliminating the need for access to challenging-to-obtain paired vision, language, and action data.
According to NVIDIA AI researcher Jim Fan, who was not involved in the paper, the innovation lies in the departure from monolithic foundation models. The research team has deconstructed the intricate task of embodied agent planning into three distinct models: a language reasoner, a visual world model, and an action planner. This approach, as Fan notes, simplifies the complex decision-making problem, rendering it more manageable and transparent. The envisaged applications for this AI system extend to aiding machines in performing household chores, such as organizing books or placing items in the dishwasher. Moreover, HiP holds promise in facilitating multistep construction and manufacturing tasks, offering assistance in stacking and arranging various materials in specific sequences.
Evaluation of HiP: AI Models Support Robots
Colorful Block Stacking Challenge: In one test, researchers instructed the robot to stack blocks of different colors and place others nearby.
HiP excelled, even when faced with the absence of some correct colors. It adeptly adapted its plans, using white blocks to paint when necessary, outperforming systems like Transformer BC and Action Diffuser.
Manipulation Task Performance: The CSAIL team subjected HiP to three manipulation tasks, demonstrating superior performance compared to similar frameworks.
HiP showcased its adaptability by developing intelligent plans that could adjust to new information, a crucial aspect of its reasoning capabilities.
Kitchen Sub-Goals Completion: In a third demonstration, HiP showcased its ability to ignore unnecessary objects while accomplishing kitchen sub-goals, such as opening a microwave, clearing a kettle, and turning on a light.
The robot efficiently adapted its plans, skipping steps that had already been completed, highlighting its practicality and efficiency in real-world scenarios.
Object Arrangement Test: Another evaluation involved arranging objects, such as candy and a hammer, in a brown box while disregarding unrelated items.
HiP demonstrated flexibility by adjusting its plans when faced with dirty objects, placing them in a cleaning box before transferring them to the designated brown container.
A Three-Tiered Approach AI Models Support Robots
The planning process of HiP operates with a three-pronged hierarchy, allowing each component to be pre-trained on diverse datasets, extending beyond the realm of robotics. Anurag Ajay, a PhD student affiliated with MIT’s Department of Electrical Engineering and Computer Science (EECS) and CSAIL, emphasizes a collaborative approach. Rather than relying on a single model to handle all tasks, the aim is to seamlessly integrate existing pre-trained models.
This collaboration involves leveraging different internet data modalities, with the combined models assisting in robotic decision-making. AI Models Support Robots, This collaborative effort holds the potential to aid tasks in various settings, including homes, factories, and construction sites. Positioned at the bottom of this hierarchy is the large language model (LLM), initiating the ideation process by capturing essential symbolic information and formulating an abstract task plan. Utilizing common sense knowledge from the internet, the LLM intelligently breaks down objectives into manageable sub-goals. An example of this is transforming the task “making a cup of tea” into specific actions like “filling a pot with water,” “boiling the pot,” and subsequent required steps.
Further More AI Models Support Robots:
- In a process called iterative refinement, HiP thinks about its ideas, taking feedback at each stage to create a more practical plan. It’s similar to how an author sends a draft to an editor, and after revisions, the final version is reviewed.
- AI models need a way to “see” and understand their surroundings to complete tasks correctly. The team used a video diffusion model to enhance the initial planning done by the large language model (LLM). This video model collects information about the world from online videos, refining the LLM’s plan to include new physical knowledge.
- At the top of the hierarchy is an egocentric action model, which uses first-person images to figure out what actions to take based on its surroundings. This helps the robot decide how to complete each task in the overall goal. For example, if a robot uses HiP to make tea, it knows where the pot, sink, and other visual elements are and starts working on each step.
However, the AI’s work is limited by the lack of high-quality video models. When available, they could improve visual prediction and robot action generation. A better version would also reduce the current data needs. Even though the CSAIL team’s method used only a small amount of data,
HiP was affordable to train and showed the potential of using existing models to finish complex tasks. According to senior author Pulkit Agrawal, this proof-of-concept demonstrates how models trained for different tasks can be combined for robotic planning. In the future, HiP could be improved with models handling touch and sound for better plans. The team is also thinking about using HiP for real-world tasks in robotics.